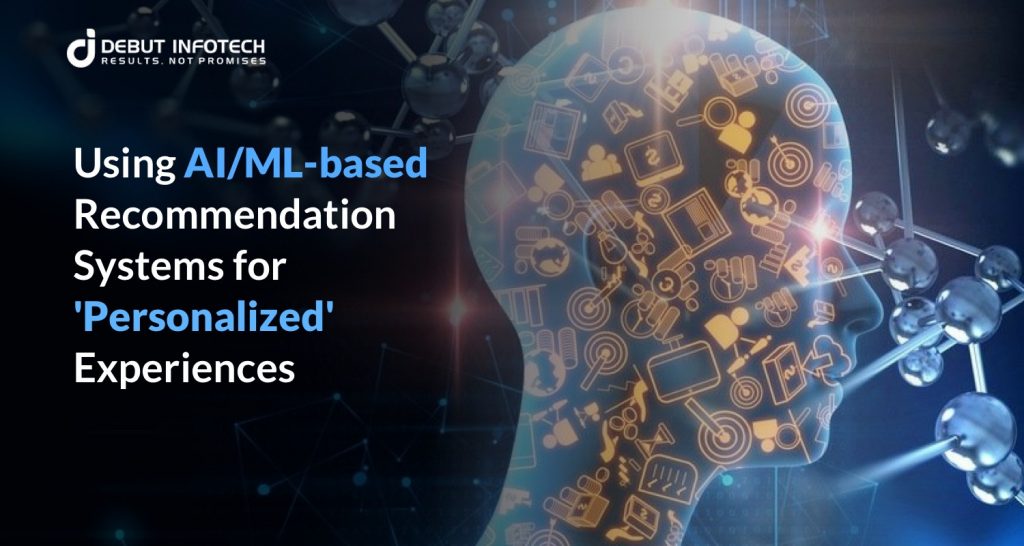
Artificial Intelligence is one of the most hyped technologies of this decade, and we all know why. From chatbots to cybersecurity systems, AI has various applications across all industries, but following the trend of personalization, AI-based recommendation systems are gaining much traction.
Now you might wonder what these systems are? An AI-based recommendation engine uses Artificial Intelligence to provide personalized recommendations to its users. These recommendations are generally derived from users’ digital behavior. In short words, ‘Personalization’ is what drives these recommendation engines.
Understanding the basics
The usage of recommendation systems is becoming an important part of the app development industry. Netflix’s “Other movies you may enjoy” and the shopping sites recommending “Customers who bought this item also bought…” are some of the best practices of the recommendation systems.
You might be surprised to know that personalization leaders such as Amazon, Netflix, etc. are witnessing a 15 to 35% increase in revenues through product recommendations.
‘Data’ is the oil that fuels recommendation systems. Want to know how? This flowchart would help you understand better:
Source: Towards Data Science
The user’s data is collected from different eCommerce platforms, search histories, websites, and social media, and is further identified and segregated to create meaningful information. The data that is collected helps you to make intelligent calculations that learn a customer’s behavior and predict what their preferences are.
This is quite similar to how humans think; for instance, you can easily guess what would be the most suitable birthday gift for your friend but the only difference here is that AI doesn’t guess and uses this data to create more logical decisions.
But how is this done? The next section describes the data processing techniques better.
Data processing techniques by AI-based recommendation systems
With such a vast amount of data available online, and so many people using this data, it becomes important as well as hard for organizations to provide consumers the data corresponding to their needs. Hence, a proper system is used by AI/ML recommendation systems to sort the data.
1. Collecting the data
As mentioned before, data is the fuel, and gathering data is one of the most important steps while creating a recommendation engine. Data can be explicit or implicit. Implicit data is collected from the users, whereas explicit data is provided by the users. Collecting the data based on the user’s behavior is not difficult; you just have to keep them logged in on your website and keep track of their likes and dislikes.
2. Storing the data
To have better recommendations, you should store the data by the algorithms you are going to use. It is completely your choice if you want to use a NoSQL database, SQL database, or any other database. A scalable and managed database reduces the number of tasks and focuses more on the recommendation itself.
3. Analyzing the data
To filter the data based on similar user engagements, it is important to filter the data with proper analyzing methodologies. Some of the ways to analyze data are as follows:
- Real-time system
To get fast recommendations, you can use this system as it processes the data the second it is created. Real-time systems consist of tools that can process and analyze event streams.
- Near real-time analysis
This method can be used for recommendations during the same browsing sessions. Near real-time analysis can collect data quickly and refresh the analytics for a few minutes, providing better data collection algorithms.
- Batch analysis
The batch analysis is beneficial when you want to create data that can be processed periodically. For example, when you want to send an email at a later date, or an analysis of daily sales volume.
4. Filtering the data
Data filtering is used to provide more relevant recommendations to the users in an artificial intelligence recommendation engine. For efficient filtering you should choose an algorithm that is suitable for the type of engine you are using:
- Content-based filtering
Content-based filtering can be used for specific shopping. The algorithms follow actions such as visited pages, items clicked on, etc. This software is developed based on products the user likes.
- Cluster
This type of filtering is connected to smaller case groups. Cluster ties two similar groups together in contrast to other types of cases and the recommended items fit each other regardless of what other users have watched or liked.
- Collaborative
This makes predictions based on the tastes and preferences of the customers. Collaborative filtering works on the idea that if two users have liked a similar item before one of them would like that again in the future.
Now, as we have understood how ‘data’ is collected, strategized, and fed to the recommendation systems, we would move forward toward some real-life practical applications of AI-based recommendation systems.
Some real-life use cases of AI-based recommendation engines
According to a survey by Bazaarvoice, only 25% of users have seen personalized home pages on the websites. However, as many as 63% of people would want to get these exclusive offers that are tailored to their preferences.
Leveraging this technology, many industries have already incorporated recommendation systems in their operations. And here are a few examples of the same:
Use-case 1: Amazon’s recommendation system – Their Secret to more sales
Looking at the success of Amazon, we can see that recommendation systems do work. Introspecting the numbers, the company reported its sales increased to $12.83 billion during its second fiscal quarter, up from $9.9 billion during the same time last year.
Amazon focuses heavily on data-driven marketing and personalization. About 35 percent of the total revenue generated by Amazon is through their recommendation systems. So, what’s their strategy?
Over the years, we all have seen how Amazon’s recommendation system works with Machine Learning. From personalized on-site recommendations to off-site emails, Amazon has its recommendation system on-point. Amazon works on item-to-item collaborative filtering across their on-site and off-site systems.
On-site recommendations provide you with recommendations from a range of services and products you have been using. Such as:
Image Source: ReJoiner
Off-site recommendations include email campaigns that send you emails based on your preferred products:
Source: ReJoiner
Use-case 2: Netflix using AI-based recommendation systems for your Netflix & Chill time
Netflix takes customer satisfaction very seriously, and to ensure the highest levels of the same, it deploys recommendation systems to provide the best results to its users. A Mckinsey study highlights that 75% of Netflix viewing is driven by recommendations.
Here are a few ways in which Netflix uses the recommendation system to bring you the best shows and movies:
1. Personalization of Movies/Shows recommendations
Using your watch history and ML-based algorithms, Netflix provides you similar shows and movies to keep you engaged.
2. Personalization of Thumbnails
Using already-built video frames from existing movies, Netflix annotates the images to identify the thumbnails which have the maximum probability of clicks from you.
3. Streaming quality
Using the viewing history data, Netflix predicts the bandwidth and caches the regional servers for faster load times during peak demand.
So, this was all for now. Many industries worldwide are using AI-based recommendation systems to provide a personalized experience to their customers. But these recommendation systems have a long way to go and still have many industries to explore.
If you are also looking for similar AI-based recommendation systems for your enterprise, we would be glad to assist you! Drop an email at [email protected] or visit us at www.debutinfotech.com to find a perfect solution for yourself!